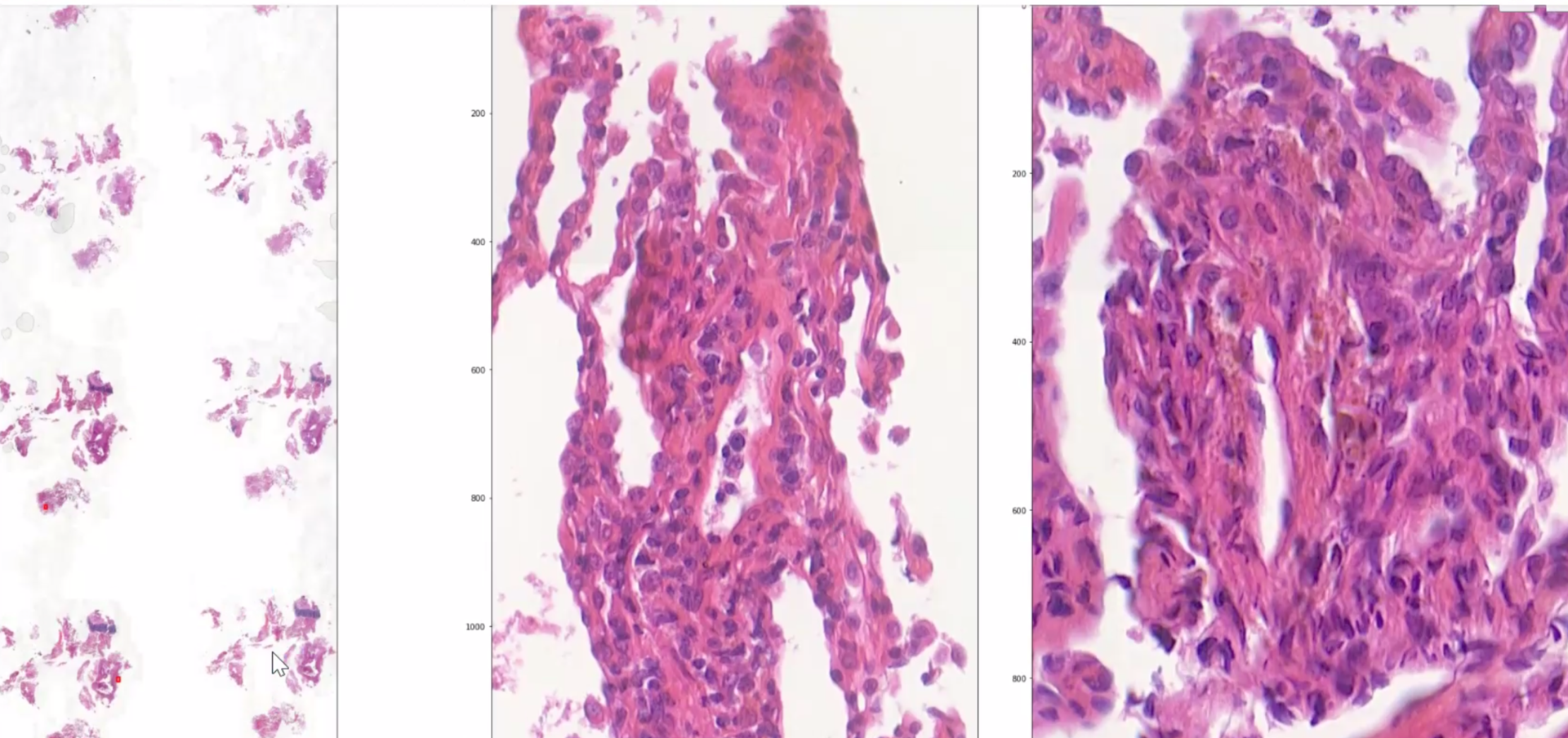
Lung transplants: 10 to 30% death rate one year after transplantation
A lung transplant is the operation of last resort for people with end-stage respiratory disease. It saves many lives, but depending on the patient profile, 10 to 30% of patients die in the year following transplantation. One of the causes of these fatal outcomes is acute transplant rejection, which requires urgent treatment. In addition, preventive biopsies of the new lung are regularly taken and submitted to the expert eye of an anatomopathologist, to detect early signs of rejection.
Loïc Le Bescond and Aymen Sadraoui, PhD students at CentraleSupélec* and part of the OPIS project team set up jointly with Inria and Paris-Saclay University in the CVN laboratory, had never worked on this subject before June 2024. But their theses dealt with a similar theme: analysis of digitised biopsies and surgical specimens using artificial intelligence tools. For Loïc, the aim was to diagnose breast cancer and understand the mechanisms of action of new treatments; for Aymen, to diagnose a particular type of liver cancer and predict the risk of recurrence.
Data Challenge: winners out of 250 participants from all over the world
Verbatim
We had expertise in analysing very large volumes of data and highly complex images. The Data Challenge, organised by Foch Hospital, was based on the same fundamental principles, with the aim of offering AI as a diagnostic aid to complement visual examination of lung biopsies.
CentraleSupélec PhD student in the OPIS team
The competition had a very high number of entrants, attracting more than 250 data scientists from all over the world. It ran from June to August 2024. "Our summer holidays were short" say the two teammates, smiling. But it was worth it: at 8 a.m. on 14 August 2024, they were declared the winners.
"Our machine learning algorithm was judged to be the most accurate and robust,” says Aymen. It is certainly a promising diagnostic aid for patient health, but it still needs improvement: “it won't be used in hospitals tomorrow: after two months, we've only just achieved proof of concept," says the researcher. “But it should now be the subject of field studies to validate and refine it, and perhaps develop clinical applications.”
The challenge: an unusual volume of data in artificial intelligence
The very short deadline of two months was a real challenge and gave rise to innovative ideas for solving a problem that a three-year PhD would not necessarily have been able to address.
Verbatim
We had a lot of ideas and not much time to explore them," says Loïc. “We had to be strategists, not just scientists. Three criteria prevailed: we focused on a proven learning model that was easy to implement and quick to train.
CentraleSupélec PhD student in the OPIS team
Developing such a model in two months was unthinkable. The teams therefore used pre-existing software components available under open-source licences, as requested by the organisers. There was a wide range to choose from; each team had to make its selection, combine the chosen components as effectively as possible, design appropriate data pre- and post-processing solutions, and manage a volume of data that is highly unusual in artificial intelligence applications.
“During a clinical trial, we analyse a few hundred digitised slides, which represents around the same number of gigabytes of data; that's already a lot," says Aymen. “For the Data Challenge, we received 4,000 digitised slides, each with 100,000 x 100,000 pixels. What's more, 2,500 of these slides were annotated by experts, to show areas that might indicate rejection. That's a total of 4 terabytes of data, the management and storage of which was a challenge in itself.”
An original approach to data pre-processing
An additional difficulty was that these areas of interest were very small compared to the size of the entire slide. Although visible, they are not sufficient to make a diagnosis straight away. It would be like trying to draw conclusions from a few microscopic needles scattered in a gigantic haystack.
The two PhD students from the OPIS team resolved this by adopting an original approach. Rather than considering each slide in its entirety, as is customary for this type of task, they cut them up into many smaller images, which they then grouped according to their size and nature (whether or not they indicated transplant rejection). As a result, the areas of interest were easier to locate and the analysis of the slide more reliable.
“The German team that came second made the same choice," says Loïc. “Which is proof that the data pre-processing was as important as the learning model itself.”
The model: a pre-trained neural network
For their model, the two PhD students chose a convolutional neural network. This type of network is designed to process and analyse images and inspired by how the visual cortex is organised in living organisms. It identifies features such as horizontal or vertical edges of images, textures, recurring patterns and so on. It then extracts the most salient patterns to best predict the solution.
Finally, it uses these characteristics to classify the images. “We started with a convolutional neural network developed to detect tumours, which classified them into four categories according to their nature, size and severity," says Aymen. “We modified it to retain only two: presence or absence of a risk of rejection.”
This (pre-trained) neural network had the advantage of having already processed a very large amount of similar data, which made it easy to apply to multiple use cases. Loïc and Aymen adapted it to the specific requirements of lung transplants, while retaining its intrinsic qualities. After the results were announced in mid-August, they submitted their code to the jury, who confirmed their win and the validity of their solution.
An auspicious start to their PhDs
Now in the final year of their PhDs, both students feel certain that they have chosen the right path, bolstered by this success. "For me, combining AI and healthcare applications is a no-brainer," says Aymen. "I can't see myself devoting years of work to a topic with no real-world relevance," says Loïc.
The two researchers do not know when or how Foch Hospital will validate their tool in the field, but they are keeping in mind the other purpose of the Data Challenge: to raise their profile as valuable scientists for research and industrial bodies that are recruiting. For them, their success in the Data Challenge already represents the start of a new story.
*They are completing their PhDs in collaboration with Gustave Roussy Institute (Loïc Le Bescond) and Bernoulli Laboratory, a joint undertaking between Inria and AP-HP (Aymen Sadraoui).
Find out more
- Data Challenges en santé : découvrez les premiers résultats du Data Challenge DigiLut ! Health Data Hub, 2/10/2024.
- Greffe pulmonaire : prévenir la dysfonction du greffon, Esinum, 13/9/2019.
- Un avatar numérique des poumons, Polytechnique Insights, 27/4/2022.