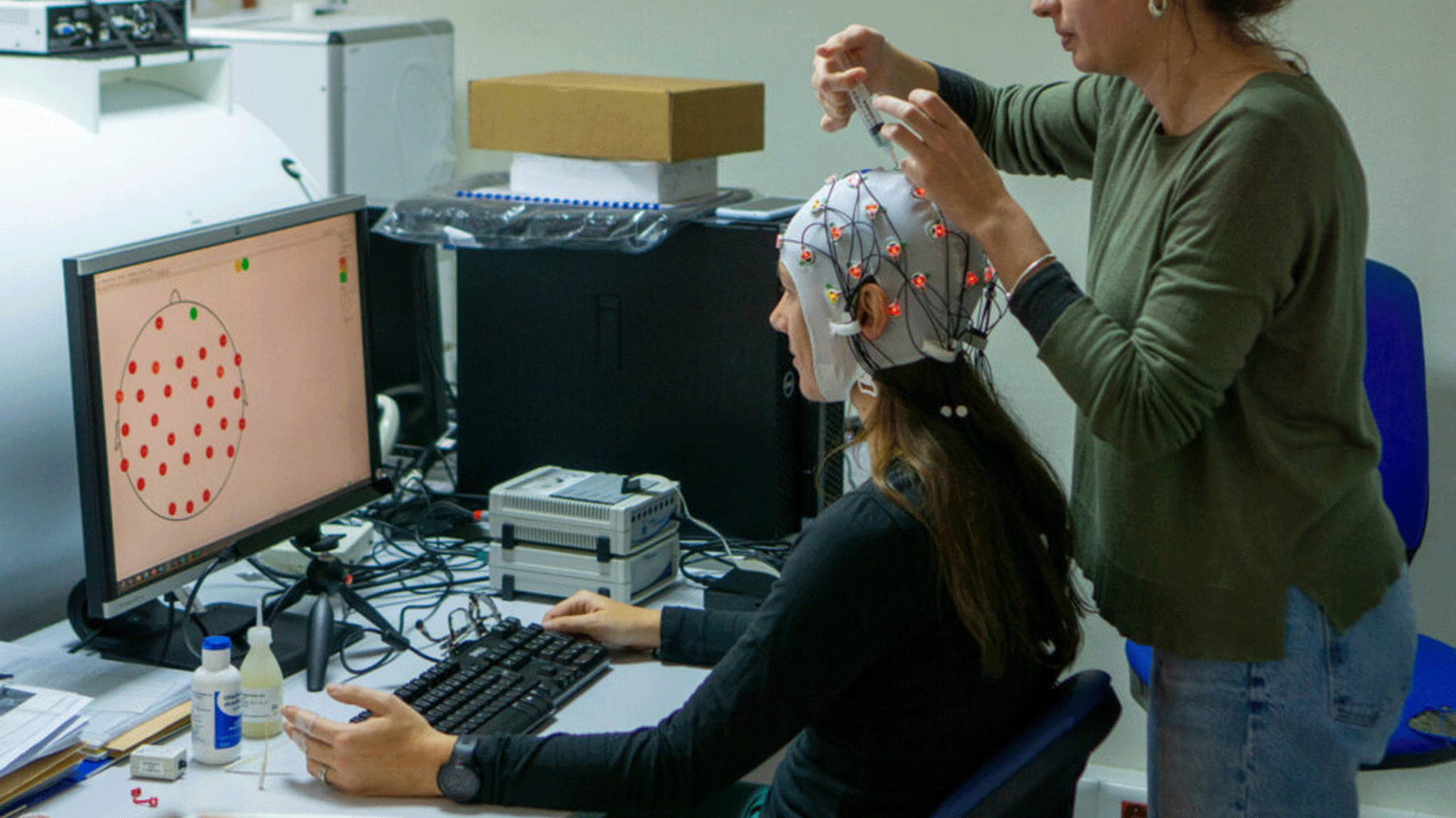
Neurofeedback is usually performed with the help of an electroencephalographic (EEG) headset. The device measures brain waves from electrodes placed on the scalp surface, while the participant is asked to perform a mental task. During this exercise, an application analyzes the brain signals and computes a score usually displayed in the form of a progress bar just like in a game. One must learn to self-regulate their brain activity to raise the bar.
In some cases, the cerebrovascular activity can also be measured by monitoring the oxygen loss due to cerebral effort. To do so, the participant must be placed in a Magnetic Resonance Imaging (MRI) tunnel with a functional sequence called fMRI. It is worth mentioning that both methods can actually be combined.
At the Inria Center at the Rennes University, the Empenn research team specializes in neuroimaging. A member of the group, scientist Claire Cury studies the processing of brain signals, in particular in the context of rehabilitation aimed at motor skill recovery after a stroke. “The problem is that when neurofeedback performance isn't good, it proves very difficult to know why, she says. There are several sources of inefficiency. The task may happen to be too demanding, thus impeding the participant to self-regulate their brain activity. Or, conversely, it may be too easy, thus prompting the participant to disengage. Or the person is simply thinking about something else. They are not doing the exercise because their mind is wandering.”
But to be fully effective, the exercise must maintain a certain level of difficulty. “Too hard, and you don’t learn. Too simple, and you don’t learn either. One must find the right balance. Except that when one starts NF training, the task is the same for everyone.”
Eye Tracking and Skin Conductance
Cury is currently leading an Inria exploratory action meant to try to identify the participant's degree of the so-called ‘task engagement’ and from there, to modulate the training target. In other words, to adjust the level of difficulty of the task according to each participant’s mental disposition at any given time. The project is called EyeSkin-NF. The key idea is to leverage two types of bio-signals that can provide information about a participant’s frame of mind. First: eye tracking. “Blink frequency, saccades amplitude and pupil diameter inform us about mental states such as cognitive fatigue or mind wandering.” Second: skin conductance. “When one gets stressed, one’s skin perspiration increases. This phenomenon has been known for a very long time. It is widely used in behavioral neuroscience —or in lie detectors.” These bio-signals also happen to be direct and thus “a priori easier to process than brain signals, which are highly complex.”
Defining Task Engagement
Conducted by post-doctoral researcher Augustina Fragueiro, a specialist in psychology and neuroscience, the first step of this Exploratory Action enabled to delineate the notion of task engagement, to identify its various facets, to devise and implement —with the help of research engineer René-Paul Debroize— a training protocol meant to generate these cognitive states of interest. From there, the following step consisted in identifying the bio-signals matching these particular frames of mind. “The protocol includes quite difficult tasks such as the Stroop exercise. People must read color names written in a different color of ink. It demands a lot of focus. There is also a very strenuous addition exercise.”
The researchers then ran a host of NF training sessions to record the bio-signals of numerous participants. They have now started analyzing the data in hope that they can really detect the mental states of interest. “We are beginning to see certain characteristics cropping up. But these findings remain very preliminary at this stage.” The results will allow to validate the protocol or to tweak it further. “Perhaps we’ll also find out that we can make do with just one modality after all, be it eye tracking or skin conductance. That would make it easier to use.”
In addition to bio-signals, the scientists also record EEG signals, which they synchronize with the other two. Initially, they planned to tap in signals from a fMRI tunnel as well. But setting up this part of the experiment turned out to be more impractical than expected. It was thus postponed.

Verbatim
What we want to do next is to check if we can measure commitment to the task not through bio-signals, but using knowledge of task engagement gained through the analysis of those bio-signals. If it works, we could then adjust the level of difficulty of neurofeedback while dispensing with eye tracking and/or skin conductance. It would make things even simpler.”
Research associate in the EMPENN project-team
In any event, once the level of task engagement has been identified, the final step would aim to upgrade the neurofeedback application so that the degree of difficulty during training would automatically adjust itself according to themental disposition of each participant all along the training session. “But we’re not there yet
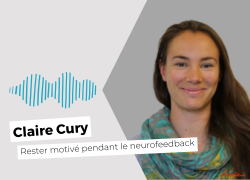
Find out more about the EyeSkin-NF project (in French)
Audio interview transcript
My name is Claire Cury and I'm a research fellow in the EMPENN team at INRIA. It's a research team that works on image and brain signal processing to aid diagnosis in general or the rehabilitation of psychiatric or neurological disorders. I'm working on neurofeedback. Neurofeedback is a technique that enables participants to learn how to self-regulate their brain activity.
There are three poles of feedback which are represented in one way or another. We represent it mainly visually. There's the pole for measuring brain activity, which is mainly done using EEG, and there's the pole for calculating the neurofeedback target. All these three poles form a loop, like a learning loop. For example, when we try to learn to play a musical instrument, we self-regulate, and when we hear that it's wrong, we change what we're doing. So that's how we learn, it's a learning loop.
In the EMPENN team, the major clinical application is post-stroke rehabilitation. In this loop, for example, the participant's objective is to recover the motor skills of his right hand. To achieve this, the neurofeedback target is calculated by measuring the brain activity corresponding to the motor region of the right hand. The problem is when neurofeedback performance isn't good. It's very difficult to know why. And there are several sources of inefficiency or non-performance in this case. For example, it could be because the task or target is too difficult. The participant is unable to self-regulate his brain activity because the target is too difficult, or because the target is too easy. So he disengages from the task, because it's too easy. Or he simply thinks about something else, and doesn't do the task. These are the cases where performance is poor.
I'm in charge of an Inria exploratory project called EyeSkin-NF, which aims to address the problem of reduced performance and therefore reduced engagement in the task by participants during neurofeedback sessions.
The idea behind the exploratory action to solve this task engagement problem was to bring in behavioral neuroscience techniques, i.e. to integrate physiological signals such as eye tracking and skin conductance, which are used quite a lot to measure wandering of the mind, attention, stress, so different cognitive states; to use these signals to then define what task engagement is based on measurements extracted from these different signals.
These signals are widely used in behavioral neuroscience. Here, we're trying to integrate them together or measure them jointly to obtain complementary information from one and the other, with EEG measurements as well.
In this exploratory action, we've set up a protocol to generate different cognitive states in the participant, and the aim is to use these cognitive states generated through the design of this protocol to measure and extract different characteristics of eye cracking and skin conductance, and to define them. So what we call task engagement.