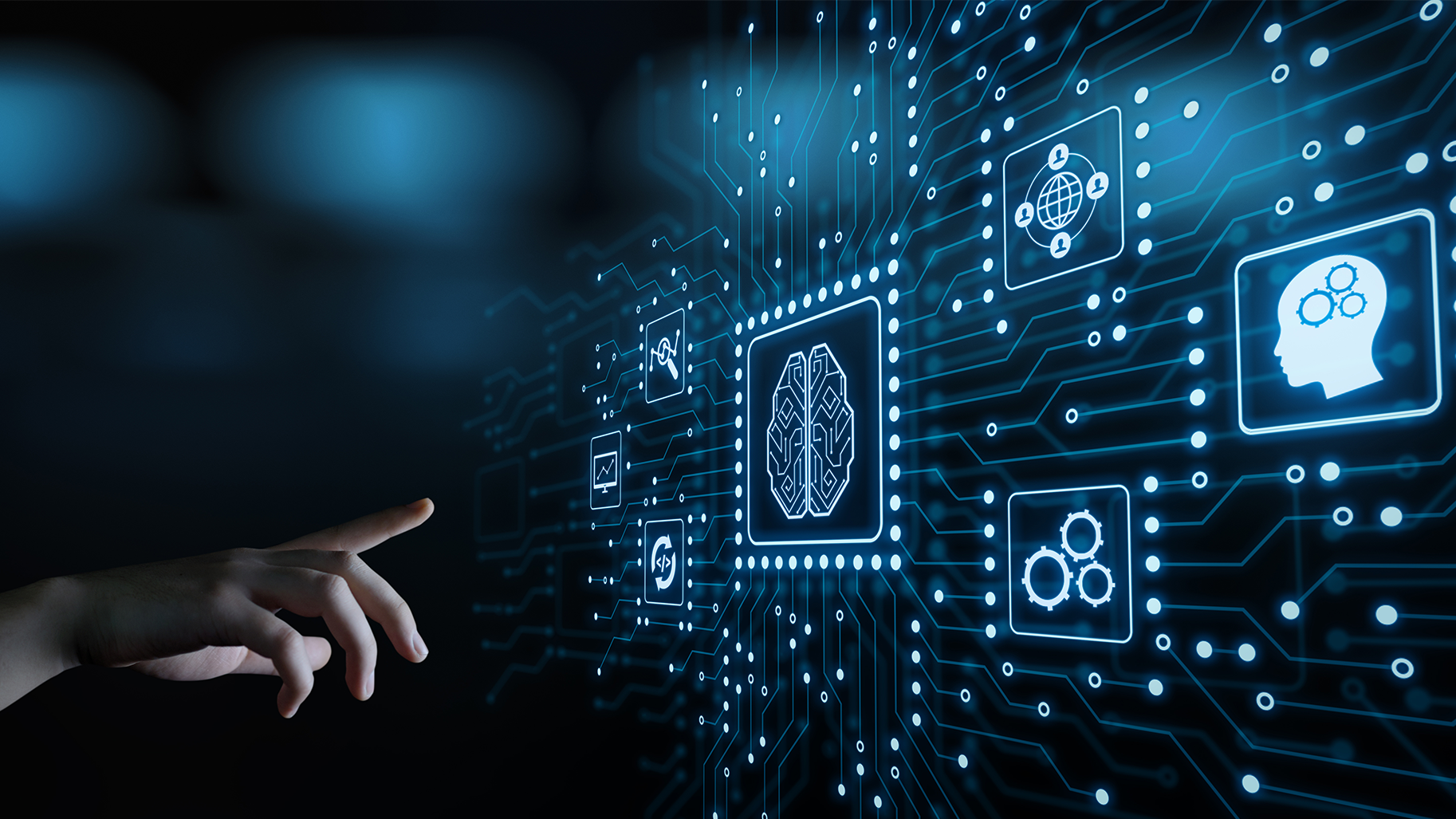
Learning consists in modifying one's behavior as a function of experience, whether for an individual or for a computer. Indeed, just like living beings, a machine can learn to modify its behavior according to its interactions with the world. While many teams at the Inria Centre at the University of Bordeaux are working on the “applications of artificial intelligence”, some are working on the "core of AI". More specifically, we are considering three particular cases of study in this situation: learning when it's hard to learn; learning to learn and reason; and making learning more adapted to human environments”, explains Frederic Alexandre, head of the Mnemosyne project-team.
Learning when it's hard to learn
For artificial intelligence, learning when it's hard to learn can pose several major challenges. One of the main reasons is the quality of the data used. If the data is noisy, corrupted, unstable, incomplete or inconsistent, this can compromise the AI's ability to draw accurate conclusions. To overcome this, scientists need to develop robust methods capable of processing large bodies of data while minimizing the environmental and financial impact. “At Potioc, for example, we are working on BCIs (Brain-Computer Interfaces), i.e. systems that translate a measurement of brain activity into commands or messages for an interactive application. For example, a BCI can recognize that a user is imagining a left or right hand movement in his brain signals, in order to move a cursor to the left or right, respectively. To do this, we process and analyze electroencephalographic (EEG) brain signals (using electrodes placed on users' heads) to try to estimate and identify the user's mental state in his EEG signals, using AI methods. Thus, if the electrodes are misplaced, if they move, if they are noisy due to electromagnetic signals (from cell phones, for example), or if the person is thinking about something other than what is required to control the system, the system will be able to detect it.
Another major challenge for AI is the lack of sufficient data. In this case, scientists can use various approaches, such as weakly supervised learning, transfer learning, or even generative models to create synthetic data. For applications to rare types of cancer, it is crucial to develop algorithms capable of operating efficiently even with a limited amount of data,” explains Olivier Saut from the Monc project team. In our team, we are developing different approaches for coupling our disease progression prediction models to clinical data. In particular, we are interested in incorporating physical constraints into the models, in task transfer, and in generative diffusion-type models for data augmentation. In all cases, the models must be sufficiently parsimonious to be interpretable.”
Finally, the progressive availability of data also poses a challenge for AI. Continuous learning, where the computer must integrate new information while retaining previously acquired knowledge, represents a complex scientific challenge. For example, the scientists in the Flowers project-team are seeking to understand, from a cognitive point of view, how humans and machines can efficiently acquire models of the world and repertoires of skills that are open and cumulative over a long period. The Mnemosyne project team, for its part, will be looking at the question from a neurobiological point of view: “The distinction between different forms of memory explains how we are able to learn autonomously over the course of our lives. At Mnemosyne, we aim to model this,” adds Frederic Alexandre.
Learning to learn and to reason
When artificial intelligence encounters learning difficulties, one promising approach is to adopt a metacognitive approach (learning how to learn). In humans, metacognition means focusing not on external elements, but on what's going on in our own minds. Metacognition involves two key steps: assessing our confidence in our own skills and knowledge, and adapting our way of thinking accordingly. By questioning our own goals and exploring creative alternatives, we can significantly improve our learning process. We model the neurobiological basis of cognitive control, i.e. how we learn to modify our behavior according to context: a process fundamental to creativity,” emphasizes Chloé Mercier, a researcher at Mnemosyne.
With the AIDE exploratory project in particular, we are using IT tools derived from knowledge representation and machine learning, to better formalize human learning and creativity as studied in the educational sciences. In other words, we seek to “translate” phenomena and concepts studied in educational science into computational terms, enabling us to apply algorithms to simulate or explain these phenomena. This formalization also enables us to dialogue more easily with colleagues in other disciplines, such as computational neuroscience. In this way, we benefit from advances in neuroscience and artificial intelligence to better understand how our children learn.
Learning to reason is also an essential aspect to be taken into account in the development of artificial intelligence. In our team, we're interested in the great adaptive potential of computerized training systems for learning at school, or for developing skills through cognitive training or rehabilitation programs when it comes to patients,” says Hélène Sauzéon, professor in the Flowers project-team. By integrating algorithms that maximize the learner's learning progress, or by establishing a dialogue with the learner in which the system invites him or her to assess his or her ability to succeed in an exercise, to plan his or her solution strategy, or to analyze the reasons for his or her successes and failures, this makes it possible to personalize learning in terms of task difficulty, while at the same time equipping the student with metacognitive tools that will enable him or her to make a lasting commitment (motivate) to learning. These synergistic loops between learning, motivation and metacognition would be effective throughout life, from infancy to old age.”
Making learning more relevant to human environments
Teams at the Inria Centre at the University of Bordeaux are also working to make learning more adapted to human environments. Several approaches are possible. It is possible to establish links with existing knowledge by calling on experts in various fields, such as the Sistm project-team, which calls on doctors to organize or exploit epidemiological data, or the Memphis project-team, which calls on notions of physics. “In the energy field, for example, we are learning to simulate different phenomena using AI combined with physical principles (flows, structures, weather), the equations that describe them and the data collected. This is particularly useful when the numerical simulation we wish to carry out requires extremely fine discretizations, resulting in excessively long calculation times,” explains Angelo Iollo, head of the Memphis project team. By combining this knowledge and expertise with learning algorithms, the scientists are able to make the results obtained more explicable. This approach enables the experts to explain the terms used, making the algorithms more understandable and acceptable to end-users.
User experience also plays a crucial role in adapting learning to human environments. For this, scientists can use natural language and multimodality; for example, the Mnemosyne project-team combines language models and brain imaging to understand the biological basis of language. Researchers can also use BCIs to assess user experience (e.g., the mental load experienced by users of a human-computer interface), such as the Potioc project-team, which seeks to estimate neuro-markers of this user experience, in users' EEG and physiological signals (the cardiac signal in particular). Finally, scientists can also take into account the social and cultural environment to make learning more adapted to human environments, as in the Flowers team, which is studying how eco-evolutionary and socio-cultural aspects can be integrated into new AI paradigms.