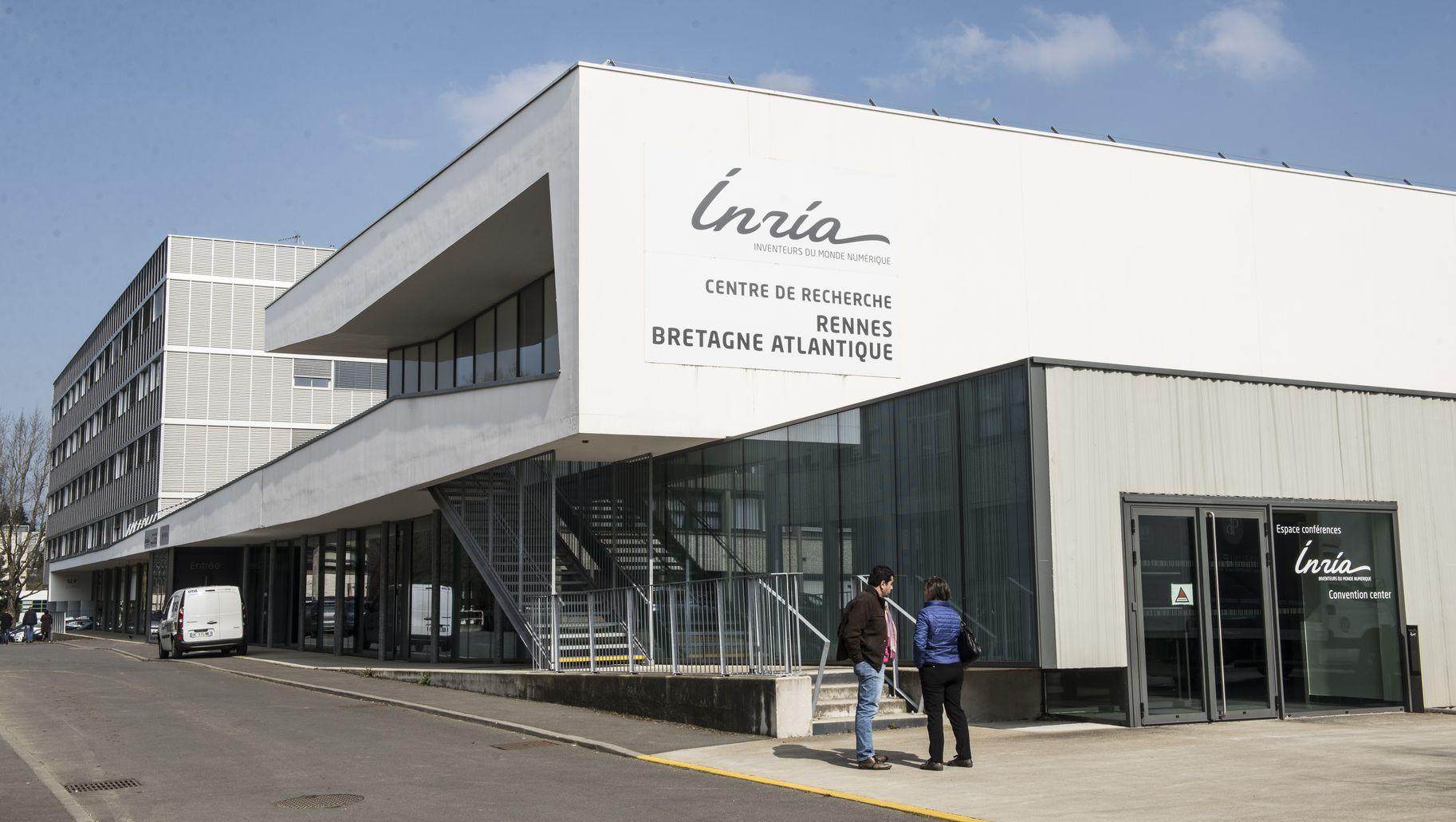
Centre Inria
de l'Université de Rennes
de l'Université de Rennes
Le centre compte 28 équipes-projets communes à Rennes (dont 24 avec l’unité mixte de recherche IRISA), 1 à Lannion. Son action mobilise plus de 600 personnes, scientifiques et personnels d’appui à la recherche et à l’innovation, issues de 50 nationalités. Le centre est également déployé à Nantes avec 2 équipes-projets communes avec le LS2N (Laboratoire des sciences du numérique de Nantes).
Vous souhaitez travailler sur des projets stimulants dans un cadre international, innovant et interdisciplinaire ? Tous les ans, Inria recrute des stagiaires, doctorants et doctorantes, postdoctorants et postdoctorantes, chargés et chargées de recherche et des directeurs ou directrices de recherche, par voie de concours ou sur contrat.
Le concours chercheurs et chercheuses 2024 est désormais clos.
Préparer ma candidature :
5 personnes ont été recrutées sur les concours 2023 au centre Inria de l'Université de Rennes.
Inria propose un environnement unique à de nombreux talents dans le domaine du développement technologique et logiciel. Le métier d’ingénieur(e) de recherche consiste à accompagner et garantir la qualité de la production de savoirs et de valeur des équipes de recherche.
Inria fait appel à des talents dans plus d’une quarantaine de métiers d'appui à la recherche allant, entre autres, des ressources humaines à l’assistanat administratif et la gestion financière en passant par la communication… Les 900 femmes et hommes des services d'appui qui accompagnent les activités de recherche et d'innovation sont une fierté de l'institut et constituent la colonne vertébrale d'Inria.
Les équipes-projets du centre sont communes avec l’unité mixte de recherche IRISA et les partenaires académiques suivants : Université de Rennes , université de Rennes 2, Nantes Université , CentraleSupelec, INSA Rennes, ENS Rennes et IMT Atlantique, CNRS, Inserm, Inrae, IFREMER, Institut Curie, Université Gustave Eiffel.
Le centre a également de nombreux autres partenariats :
Adresse
Centre Inria de l'Université de Rennes
Campus universitaire de Beaulieu
Avenue du Général Leclerc
35042 Rennes Cedex
Contacts
Nos autres sites :